09
MayAzure Synapse Vs. Databricks
If you’re diving into data analytics or big data projects, you’ve probably heard about Azure Synapse Vs. Databricks. They’re both powerful platforms, but they’re built for different types of work. Azure Synapse is great for things like data integration and business reporting, while Databricks is more focused on big data processing, AI, and real-time analytics.
In this Azure tutorial, we’ll help you decide which platform is right for your project Azure Synapse or Azure Databricks. We’ll break down the core differences, highlight what each tool does best, and explain when to use them. Whether you're working with massive datasets, building machine learning models, or simply organizing data efficiently, this comparison will guide you toward the right choice.
What is Azure Synapse?
Azure Synapse Analytics is a cloud service from Microsoft Azure that helps you analyze and visualize data. It combines big data and data warehousing, allowing you to work with data from different sources. With Azure Synapse, you can run real-time analytics, explore your data, and gain business insights, making it easier to manage and use your data for smarter decisions.
Features of Azure Synapse
Azure Synapse offers powerful features to streamline data management and analytics. Here are its key capabilities:
1. Unified platform for data integration, warehousing, and big data analytics.
2. Support for serverless and provisioned compute options:
Azure Synapse offers both serverless and provisioned compute options, giving you the flexibility to choose the best approach for your workload. Serverless compute allows you to pay only for the resources you use, while provisioned compute offers dedicated resources for more consistent performance and scalability. This flexibility helps optimize costs and performance based on your specific needs.
3. Integration with other Azure services:
Azure Synapse seamlessly integrates with other Azure services, such as Azure Machine Learning, Azure Data Lake, and Power BI, enabling a smooth data flow across the platform. This integration enhances your ability to analyze data, build machine learning models, and create interactive reports, all within a unified environment.
Read More: |
4. Built-in data exploration and visualization tools:
Azure Synapse provides built-in data exploration and visualization tools that allow users to easily interact with and analyze their data. These tools enable quick insights through intuitive dashboards and reports, making it simple to uncover trends and patterns without needing additional software or complex setups.
5. Security and compliance features:
Azure Synapse offers robust security and compliance features to protect your data. It includes advanced encryption, role-based access control, and integration with Azure Active Directory for identity management. These features ensure that your data is secure, meets industry standards, and complies with regulations such as GDPR and HIPAA.
Read More: Top Azure Active Directory Interview Questions for 2025 |
What is Databricks?
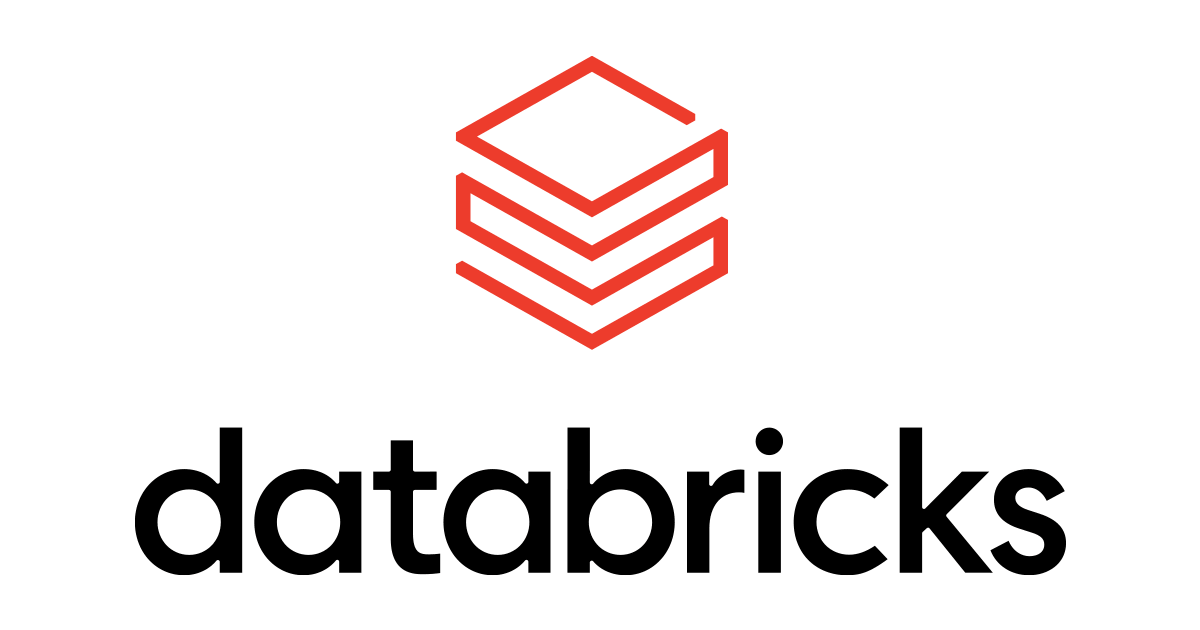
Features of Databricks
Databricks offers a variety of powerful features that streamline data engineering, analytics, and machine learning. Here are some of its key capabilities:
1. High-performance data processing with Apache Spark:
Databricks provides high-performance data processing with Apache Spark, allowing users to process large volumes of data quickly and efficiently. This enables real-time analytics and machine learning at scale, making it ideal for handling complex data workflows and accelerating data-driven decision-making.
2. Collaborative notebooks for data science and machine learning:
Databricks offers collaborative notebooks that enable data science and machine learning teams to work together seamlessly. These notebooks support interactive coding, visualization, and real-time collaboration, making it easier to share insights, experiment with models, and track progress in a unified environment.
3. Integration with a wide range of data sources, including Delta Lake:
Databricks provides seamless integration with a wide range of data sources, including Delta Lake. This allows users to easily ingest, store, and manage structured and unstructured data while ensuring data reliability, consistency, and high performance across various data environments.
4. Advanced analytics and AI/ML capabilities:
Databricks offers advanced analytics and AI/ML capabilities that empower teams to build, train, and deploy machine learning models at scale. With built-in tools for data exploration, model training, and real-time analytics, Databricks simplifies the end-to-end process of creating AI-powered solutions and driving data-driven insights.
5. Scalability and performance optimization:
Databricks ensures scalability and performance optimization by leveraging Apache Spark's distributed computing capabilities. It automatically adjusts resources to meet workload demands, allowing for high performance even with large datasets. This flexibility enables users to scale their data processing and analytics without compromising on speed or efficiency.
Azure Synapse vs Databricks: Main Differences
1. Purpose and use cases
2. Data integration and ETL capabilities
3. Analytics and machine learning
4. Performance and scalability
5. Integration with other Azure services
6. User experience and ease of use
7. Cost considerations
Azure Synapse vs Databricks: A Summary
Category | Azure Synapse Analytics | Databricks |
Overview | Integrated analytics service for big data and data warehousing. | Unified platform for big data and machine learning. |
Primary Use Case | Data warehousing, big data analytics, and data integration. | Big data processing, data science, and machine learning. |
Data Integration | Built-in data integration (Synapse Pipelines). | Requires Azure Data Factory for data pipelines. |
Compute Engine | SQL-based compute engine (serverless and provisioned). | Apache Spark-based compute engine for big data. |
Scalability | Flexible, on-demand, and provisioned options. | Auto-scaling clusters for big data processing. |
Machine Learning | Integrated with Azure Machine Learning. | Built-in support for ML with MLflow and Spark MLlib. |
Notebooks | Integrated notebooks supporting SQL, Python, Scala, and R. | Advanced notebooks for Python, Scala, SQL, and R. |
Cost Model | Pay-as-you-go for storage and compute. | Pay-as-you-go for storage and compute. |
Security | Role-based access, encryption, and private endpoints. | Encryption, AAD integration, and access controls. |
Ease of Use | User-friendly, with drag-and-drop features. | More technical, suited for data scientists and engineers. |
When to Use Azure Synapse
Azure Synapse vs. Databricks is often discussed when choosing the right tool for specific analytics needs. When to use Azure Synapse depends on several key scenarios:
- Data Warehousing and Big Data Analytics: Ideal for managing and analyzing large volumes of structured and unstructured data.
- ETL Processes: Streamline data integration and transformation using Synapse Pipelines.
- Real-time Analytics: Process real-time data streams with integrations like Azure Stream Analytics.
- Business Intelligence: Easily integrates with Power BI to visualize complex data insights.
In the Azure Synapse vs. Databricks comparison, Synapse shines when you need unified analytics within the Azure ecosystem and a strong BI integration.
When to Use Databricks
Azure Synapse vs. Databricks is a frequent comparison for modern data teams evaluating analytics platforms. When to use Databricks depends on the following key scenarios:
- Big Data Processing: When you need to process and analyze large-scale datasets using Apache Spark.
- Machine Learning and AI: Ideal for building, training, and deploying machine learning models, with built-in support for MLflow and Spark MLlib.
- Collaborative Data Science: Perfect for teams working together on notebooks and data exploration, supporting languages like Python, Scala, SQL, and R.
- Delta Lake Integration: When you need high-performance storage with ACID transaction support for big data workloads.
In the Azure Synapse vs. Databricks comparison, Databricks stands out for advanced machine learning and large-scale data science collaboration.
Summary:
Azure Synapse is a comprehensive analytics service ideal for data integration, warehousing, and running large-scale analytics with SQL-based tools, making it perfect for organizations focusing on data reporting and business intelligence. On the other hand, Databricks is built on Apache Spark and excels in big data processing, machine learning, and real-time analytics, making it the go-to platform for data scientists and engineers working with advanced analytics and AI. While Synapse is suited for structured data and business intelligence, Databricks shines in machine learning and data engineering projects, offering strong capabilities for those working with large datasets and complex algorithms.
Read More: |
Let’s find out: Azure Synapse Vs. Databricks
Q 1: What is Azure Synapse primarily used for?
Take our Azure skill challenge to evaluate yourself!
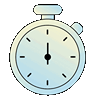
In less than 5 minutes, with our skill challenge, you can identify your knowledge gaps and strengths in a given skill.